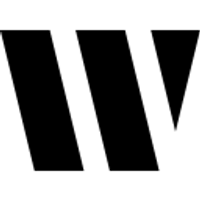
Writer/Palmyra-local-1_7B
Updated
β’
11
β’
1
AGI, LLMs, Knowledge Graph, Palmyra, Domain Specific LLM
meta-llama/Llama-3.2-1B
adapt in real time using user feedback. Check out the code and white paper on GitHub!transformers
:pip install transformers torch numpy
python Adaptive_Learning_(GESAL).py