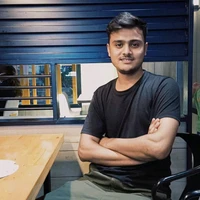
mahwizzzz/UrduIntentClassification
Text Classification
β’
Updated
β’
8
id
string | label
string | label_text
string | text
string | lang
string |
---|---|---|---|---|
1 | alarm_set | alarm_set | maak my wakker nege-uur v. m. op vrydag | af |
2 | alarm_set | alarm_set | stel 'n alarm vir twee ure van nou af | af |
4 | audio_volume_mute | audio_volume_mute | janneman stilte | af |
5 | audio_volume_mute | audio_volume_mute | stop | af |
6 | audio_volume_mute | audio_volume_mute | janneman onderbreek dit vir tien sekondes | af |
7 | audio_volume_mute | audio_volume_mute | stop vir tien sekondes | af |
9 | iot_hue_lightchange | iot_hue_lightchange | maak die beligting bietjie meer warm hier | af |
10 | iot_hue_lightchange | iot_hue_lightchange | stel asseblief die beligting geskik vir lees | af |
12 | iot_hue_lightoff | iot_hue_lightoff | tyd om te slaap | af |
13 | iot_hue_lightoff | iot_hue_lightoff | tyd om te slaap janneman | af |
15 | iot_hue_lightoff | iot_hue_lightoff | skakel af die lig in die badkamer | af |
16 | iot_hue_lightdim | iot_hue_lightdim | olly verdof die ligte in die gang | af |
18 | iot_hue_lightoff | iot_hue_lightoff | skakel die ligte af in die slaapkamer | af |
20 | iot_hue_lightchange | iot_hue_lightchange | stel die ligte na twintig persent | af |
21 | iot_hue_lightchange | iot_hue_lightchange | olly stel ligte tot twintig persent | af |
22 | iot_hue_lightdim | iot_hue_lightdim | olly verdof die ligte in die kombuis | af |
23 | iot_hue_lightdim | iot_hue_lightdim | verdof die ligte in die kombuis | af |
25 | iot_cleaning | iot_cleaning | janneman maak die woonstel skoon | af |
28 | iot_cleaning | iot_cleaning | stofsuig die huis | af |
29 | iot_cleaning | iot_cleaning | stofsuig die huis olly | af |
32 | iot_cleaning | iot_cleaning | stofsuig die matte | af |
33 | calendar_query | calendar_query | kyk wanneer die vertoning begin | af |
34 | play_music | play_music | ek will weer na 'n koos kombuis liedjie luister | af |
35 | play_music | play_music | ek wil weer daardie musiek een speel | af |
36 | general_quirky | general_quirky | kyk my motor is gereed | af |
37 | general_quirky | general_quirky | kyk of my skootrekenaar werk | af |
38 | general_quirky | general_quirky | is die helderheid van my skerm laag | af |
39 | general_quirky | general_quirky | ek moet liggingsdienste aan hΓͺ kan jy kyk | af |
40 | general_quirky | general_quirky | kyk die status van my krag verbruik | af |
43 | general_quirky | general_quirky | ek is nie moeg nie ek is eintlik gelukkig | af |
44 | general_quirky | general_quirky | ollie ek is nie moeg nie ek is eintlik gelukkig | af |
45 | general_greet | general_greet | wat gaan aan | af |
48 | datetime_query | datetime_query | vertel my die tyd in moskou | af |
49 | datetime_convert | datetime_convert | se vir my die tyd g. m. t. plus vyf | af |
51 | takeaway_query | takeaway_query | olly lys mees gegradeerde afleweringsopsies vir sjinese kos | af |
52 | takeaway_query | takeaway_query | mees gegradeerde aflewering opsies vir chinese kos | af |
54 | takeaway_query | takeaway_query | olly hoogste gegradeerde aflewerings opsies vir chinese kos | af |
55 | takeaway_query | takeaway_query | ek wil kerrie as wegneem hΓͺ enige aanbevelings | af |
56 | takeaway_query | takeaway_query | ek wil 'n bietjie kerrie hΓͺ om te gaan enige aanbevelings janneman | af |
57 | takeaway_query | takeaway_query | vind my taiwannese wegneemetes rondom die waterfront | af |
60 | alarm_remove | alarm_remove | stop sewe-uur v. m. alarm | af |
62 | alarm_query | alarm_query | lys asseblief aktiewe alarms | af |
65 | news_query | news_query | wat gebeur vandag in rugby | af |
66 | play_music | play_music | speel asseblief yesterday van die beatles | af |
69 | music_likeness | music_likeness | ek hou van rock musiek | af |
70 | music_likeness | music_likeness | my gunsteling musiek groep is queen | af |
72 | play_music | play_music | begin musiek van nadine speel | af |
73 | play_music | play_music | speel asseblief my beste musiek | af |
75 | music_query | music_query | wie het die musiek wat nou speel gekomponeer | af |
76 | music_query | music_query | wat is daardie die album is huidige musiek van | af |
77 | music_likeness | music_likeness | olly ek geniet regtig hierdie liedjie | af |
78 | music_likeness | music_likeness | die lied wat jy speel is wonderlik | af |
79 | music_likeness | music_likeness | dit is een van die beste liedjies vir my | af |
81 | iot_hue_lightup | iot_hue_lightup | maak ligte helderder | af |
82 | iot_hue_lightup | iot_hue_lightup | verhoog asseblief die ligte voluit | af |
83 | iot_cleaning | iot_cleaning | het skakel die stofsuier robot aan | af |
84 | iot_cleaning | iot_cleaning | skakel die skoonmaker robot aan | af |
87 | takeaway_order | takeaway_order | bestel asseblief soesji vir aandete | af |
88 | takeaway_order | takeaway_order | haai ek wil hΓͺ jy moet 'n burger bestel | af |
91 | takeaway_order | takeaway_order | kan ek wegneem aandete bestel van romans | af |
92 | takeaway_query | takeaway_query | doen biesmiellah wegneem etes | af |
93 | alarm_set | alarm_set | stel 'n wekker vir twaalf | af |
94 | alarm_set | alarm_set | stel 'n alarm veertig minute van nou af | af |
95 | alarm_set | alarm_set | stel alarm vir agtuur elke weeksdag | af |
96 | weather_query | weather_query | reΓ«n dit | af |
97 | weather_query | weather_query | gaan dit reΓ«n | af |
98 | weather_query | weather_query | sneeu dit tans | af |
101 | weather_query | weather_query | wat is die week se weer | af |
104 | news_query | news_query | vertel my b. b. c. nuus | af |
105 | news_query | news_query | wat is die nuss op b. b. c. nuus | af |
106 | news_query | news_query | wat is e nuus se nuutste nuus | af |
108 | play_music | play_music | speel 'n liedjie waarvan ek hou | af |
110 | play_music | play_music | speel daft punk | af |
111 | play_music | play_music | sit aan 'n bietjie coldplay | af |
114 | music_settings | music_settings | skommel hierdie speellys | af |
116 | music_query | music_query | wat speel nou | af |
117 | music_query | music_query | watter musiek is hierdie | af |
118 | music_query | music_query | sΓͺ my die kunstenaar van die song | af |
119 | general_joke | general_joke | maak my lag | af |
120 | general_joke | general_joke | janneman laat my lag | af |
121 | general_joke | general_joke | vertel my n goeie grappie | af |
123 | general_joke | general_joke | vertel my 'n grap | af |
125 | general_joke | general_joke | alexa vertel vir my n grappie | af |
126 | general_joke | general_joke | kikker my op | af |
129 | general_quirky | general_quirky | vertel my van vandag | af |
130 | takeaway_order | takeaway_order | bestel 'n pizza | af |
131 | takeaway_order | takeaway_order | bestel vir my 'n boerie rolletjie van bo-kaap kombuis | af |
133 | takeaway_query | takeaway_query | wanneer kom my bestelling | af |
134 | takeaway_query | takeaway_query | hoe lank nog vir my wegneem ete | af |
135 | takeaway_query | takeaway_query | domino's aflewering status | af |
136 | music_query | music_query | wat speel nou | af |
137 | music_query | music_query | sΓͺ vir my die liedjie se naam | af |
139 | play_music | play_music | speel my jazz speel lys | af |
140 | play_music | play_music | begin my jazz speellys | af |
141 | play_music | play_music | speel my gunsteling speellys | af |
142 | music_likeness | music_likeness | dit is a goeie lied | af |
143 | music_dislikeness | music_dislikeness | ek hou nie daarvan nie | af |
144 | music_likeness | music_likeness | ek hou daarvan | af |
145 | music_likeness | music_likeness | ek hou van jazz | af |
146 | play_music | play_music | kan jy bietjie jazz speel | af |
MASSIVE: A 1M-Example Multilingual Natural Language Understanding Dataset with 51 Typologically-Diverse Languages
Task category | t2c |
Domains | Spoken |
Reference | https://arxiv.org/abs/2204.08582 |
You can evaluate an embedding model on this dataset using the following code:
import mteb
task = mteb.get_tasks(["MassiveIntentClassification"])
evaluator = mteb.MTEB(task)
model = mteb.get_model(YOUR_MODEL)
evaluator.run(model)
To learn more about how to run models on mteb
task check out the GitHub repitory.
If you use this dataset, please cite the dataset as well as mteb, as this dataset likely includes additional processing as a part of the MMTEB Contribution.
@misc{fitzgerald2022massive,
archiveprefix = {arXiv},
author = {Jack FitzGerald and Christopher Hench and Charith Peris and Scott Mackie and Kay Rottmann and Ana Sanchez and Aaron Nash and Liam Urbach and Vishesh Kakarala and Richa Singh and Swetha Ranganath and Laurie Crist and Misha Britan and Wouter Leeuwis and Gokhan Tur and Prem Natarajan},
eprint = {2204.08582},
primaryclass = {cs.CL},
title = {MASSIVE: A 1M-Example Multilingual Natural Language Understanding Dataset with 51 Typologically-Diverse Languages},
year = {2022},
}
@article{enevoldsen2025mmtebmassivemultilingualtext,
title={MMTEB: Massive Multilingual Text Embedding Benchmark},
author={Kenneth Enevoldsen and Isaac Chung and Imene Kerboua and MΓ‘rton Kardos and Ashwin Mathur and David Stap and Jay Gala and Wissam Siblini and Dominik KrzemiΕski and Genta Indra Winata and Saba Sturua and Saiteja Utpala and Mathieu Ciancone and Marion Schaeffer and Gabriel Sequeira and Diganta Misra and Shreeya Dhakal and Jonathan RystrΓΈm and Roman Solomatin and Γmer ΓaΔatan and Akash Kundu and Martin Bernstorff and Shitao Xiao and Akshita Sukhlecha and Bhavish Pahwa and RafaΕ PoΕwiata and Kranthi Kiran GV and Shawon Ashraf and Daniel Auras and BjΓΆrn PlΓΌster and Jan Philipp Harries and LoΓ―c Magne and Isabelle Mohr and Mariya Hendriksen and Dawei Zhu and Hippolyte Gisserot-Boukhlef and Tom Aarsen and Jan Kostkan and Konrad Wojtasik and Taemin Lee and Marek Ε uppa and Crystina Zhang and Roberta Rocca and Mohammed Hamdy and Andrianos Michail and John Yang and Manuel Faysse and Aleksei Vatolin and Nandan Thakur and Manan Dey and Dipam Vasani and Pranjal Chitale and Simone Tedeschi and Nguyen Tai and Artem Snegirev and Michael GΓΌnther and Mengzhou Xia and Weijia Shi and Xing Han LΓΉ and Jordan Clive and Gayatri Krishnakumar and Anna Maksimova and Silvan Wehrli and Maria Tikhonova and Henil Panchal and Aleksandr Abramov and Malte Ostendorff and Zheng Liu and Simon Clematide and Lester James Miranda and Alena Fenogenova and Guangyu Song and Ruqiya Bin Safi and Wen-Ding Li and Alessia Borghini and Federico Cassano and Hongjin Su and Jimmy Lin and Howard Yen and Lasse Hansen and Sara Hooker and Chenghao Xiao and Vaibhav Adlakha and Orion Weller and Siva Reddy and Niklas Muennighoff},
publisher = {arXiv},
journal={arXiv preprint arXiv:2502.13595},
year={2025},
url={https://arxiv.org/abs/2502.13595},
doi = {10.48550/arXiv.2502.13595},
}
@article{muennighoff2022mteb,
author = {Muennighoff, Niklas and Tazi, Nouamane and Magne, Lo{\"\i}c and Reimers, Nils},
title = {MTEB: Massive Text Embedding Benchmark},
publisher = {arXiv},
journal={arXiv preprint arXiv:2210.07316},
year = {2022}
url = {https://arxiv.org/abs/2210.07316},
doi = {10.48550/ARXIV.2210.07316},
}
The following code contains the descriptive statistics from the task. These can also be obtained using:
import mteb
task = mteb.get_task("MassiveIntentClassification")
desc_stats = task.metadata.descriptive_stats
{
"validation": {
"num_samples": 103683,
"number_of_characters": 3583467,
"number_texts_intersect_with_train": 5457,
"min_text_length": 1,
"average_text_length": 34.56176036573016,
"max_text_length": 224,
"unique_text": 102325,
"unique_labels": 59,
"labels": {
"iot_hue_lightoff": {
"count": 867
},
"iot_hue_lightdim": {
"count": 867
},
"iot_cleaning": {
"count": 969
},
"general_quirky": {
"count": 5355
},
"takeaway_query": {
"count": 1224
},
"play_music": {
"count": 6273
},
"music_query": {
"count": 1530
},
"weather_query": {
"count": 6426
},
"music_settings": {
"count": 408
},
"audio_volume_down": {
"count": 408
},
"datetime_query": {
"count": 3264
},
"general_greet": {
"count": 102
},
"alarm_set": {
"count": 1581
},
"audio_volume_up": {
"count": 612
},
"alarm_query": {
"count": 969
},
"news_query": {
"count": 4182
},
"iot_hue_lighton": {
"count": 255
},
"iot_wemo_off": {
"count": 255
},
"iot_hue_lightchange": {
"count": 1122
},
"audio_volume_mute": {
"count": 765
},
"alarm_remove": {
"count": 714
},
"general_joke": {
"count": 765
},
"datetime_convert": {
"count": 459
},
"iot_wemo_on": {
"count": 357
},
"iot_hue_lightup": {
"count": 612
},
"iot_coffee": {
"count": 714
},
"social_post": {
"count": 2550
},
"music_dislikeness": {
"count": 102
},
"cooking_recipe": {
"count": 2091
},
"takeaway_order": {
"count": 1020
},
"music_likeness": {
"count": 816
},
"calendar_query": {
"count": 5202
},
"qa_stock": {
"count": 1224
},
"qa_factoid": {
"count": 4590
},
"calendar_set": {
"count": 6681
},
"recommendation_events": {
"count": 1326
},
"cooking_query": {
"count": 102
},
"calendar_remove": {
"count": 2397
},
"email_sendemail": {
"count": 3213
},
"play_radio": {
"count": 2346
},
"play_audiobook": {
"count": 1785
},
"play_game": {
"count": 1122
},
"lists_query": {
"count": 2550
},
"lists_remove": {
"count": 1887
},
"lists_createoradd": {
"count": 1275
},
"email_addcontact": {
"count": 255
},
"play_podcasts": {
"count": 1734
},
"recommendation_movies": {
"count": 612
},
"recommendation_locations": {
"count": 1581
},
"transport_ticket": {
"count": 1275
},
"transport_query": {
"count": 1836
},
"transport_taxi": {
"count": 1377
},
"transport_traffic": {
"count": 1122
},
"qa_definition": {
"count": 2805
},
"qa_currency": {
"count": 1632
},
"qa_maths": {
"count": 663
},
"social_query": {
"count": 918
},
"email_query": {
"count": 3723
},
"email_querycontact": {
"count": 816
}
}
},
"test": {
"num_samples": 151674,
"number_of_characters": 5230011,
"number_texts_intersect_with_train": 7273,
"min_text_length": 1,
"average_text_length": 34.48192175323391,
"max_text_length": 495,
"unique_text": 148972,
"unique_labels": 59,
"labels": {
"alarm_set": {
"count": 2091
},
"audio_volume_mute": {
"count": 1632
},
"iot_hue_lightchange": {
"count": 1836
},
"iot_hue_lighton": {
"count": 153
},
"iot_hue_lightoff": {
"count": 2193
},
"iot_cleaning": {
"count": 1326
},
"general_quirky": {
"count": 8619
},
"general_greet": {
"count": 51
},
"datetime_query": {
"count": 4488
},
"datetime_convert": {
"count": 765
},
"alarm_remove": {
"count": 1071
},
"alarm_query": {
"count": 1734
},
"music_likeness": {
"count": 1836
},
"iot_hue_lightup": {
"count": 1377
},
"takeaway_order": {
"count": 1122
},
"weather_query": {
"count": 7956
},
"general_joke": {
"count": 969
},
"play_music": {
"count": 8976
},
"iot_hue_lightdim": {
"count": 1071
},
"takeaway_query": {
"count": 1785
},
"news_query": {
"count": 6324
},
"audio_volume_up": {
"count": 663
},
"iot_wemo_off": {
"count": 918
},
"iot_wemo_on": {
"count": 510
},
"iot_coffee": {
"count": 1836
},
"music_query": {
"count": 1785
},
"audio_volume_down": {
"count": 561
},
"audio_volume_other": {
"count": 306
},
"music_dislikeness": {
"count": 204
},
"music_settings": {
"count": 306
},
"recommendation_events": {
"count": 2193
},
"qa_stock": {
"count": 1326
},
"calendar_set": {
"count": 10659
},
"play_audiobook": {
"count": 2091
},
"social_query": {
"count": 1275
},
"qa_factoid": {
"count": 7191
},
"transport_ticket": {
"count": 1785
},
"recommendation_locations": {
"count": 1581
},
"calendar_query": {
"count": 6426
},
"recommendation_movies": {
"count": 1020
},
"transport_query": {
"count": 2601
},
"cooking_recipe": {
"count": 3672
},
"play_game": {
"count": 1785
},
"calendar_remove": {
"count": 3417
},
"email_query": {
"count": 6069
},
"email_sendemail": {
"count": 5814
},
"play_radio": {
"count": 3672
},
"play_podcasts": {
"count": 3213
},
"lists_query": {
"count": 2601
},
"lists_remove": {
"count": 2652
},
"lists_createoradd": {
"count": 1989
},
"transport_taxi": {
"count": 1173
},
"transport_traffic": {
"count": 765
},
"qa_definition": {
"count": 2907
},
"qa_maths": {
"count": 1275
},
"social_post": {
"count": 4131
},
"qa_currency": {
"count": 1989
},
"email_addcontact": {
"count": 612
},
"email_querycontact": {
"count": 1326
}
}
},
"train": {
"num_samples": 587214,
"number_of_characters": 20507758,
"number_texts_intersect_with_train": null,
"min_text_length": 1,
"average_text_length": 34.92382334208653,
"max_text_length": 295,
"unique_text": 565055,
"unique_labels": 60,
"labels": {
"alarm_set": {
"count": 9282
},
"audio_volume_mute": {
"count": 5610
},
"iot_hue_lightchange": {
"count": 6375
},
"iot_hue_lightoff": {
"count": 7803
},
"iot_hue_lightdim": {
"count": 3876
},
"iot_cleaning": {
"count": 4743
},
"calendar_query": {
"count": 28866
},
"play_music": {
"count": 32589
},
"general_quirky": {
"count": 28305
},
"general_greet": {
"count": 1275
},
"datetime_query": {
"count": 17850
},
"datetime_convert": {
"count": 2652
},
"takeaway_query": {
"count": 6222
},
"alarm_remove": {
"count": 3978
},
"alarm_query": {
"count": 6630
},
"news_query": {
"count": 25653
},
"music_likeness": {
"count": 5763
},
"music_query": {
"count": 7854
},
"iot_hue_lightup": {
"count": 3876
},
"takeaway_order": {
"count": 6885
},
"weather_query": {
"count": 29223
},
"music_settings": {
"count": 2601
},
"general_joke": {
"count": 3672
},
"music_dislikeness": {
"count": 714
},
"audio_volume_other": {
"count": 918
},
"iot_coffee": {
"count": 6324
},
"audio_volume_up": {
"count": 5610
},
"iot_wemo_on": {
"count": 2448
},
"iot_hue_lighton": {
"count": 1122
},
"iot_wemo_off": {
"count": 2652
},
"audio_volume_down": {
"count": 2652
},
"qa_stock": {
"count": 7752
},
"play_radio": {
"count": 14433
},
"recommendation_locations": {
"count": 8823
},
"qa_factoid": {
"count": 27744
},
"calendar_set": {
"count": 41310
},
"play_audiobook": {
"count": 7650
},
"play_podcasts": {
"count": 9843
},
"social_query": {
"count": 5508
},
"transport_query": {
"count": 11577
},
"email_sendemail": {
"count": 18054
},
"recommendation_movies": {
"count": 3570
},
"lists_query": {
"count": 10098
},
"play_game": {
"count": 5712
},
"transport_ticket": {
"count": 6477
},
"recommendation_events": {
"count": 9690
},
"email_query": {
"count": 21318
},
"transport_traffic": {
"count": 5967
},
"cooking_query": {
"count": 204
},
"qa_definition": {
"count": 13617
},
"calendar_remove": {
"count": 15912
},
"lists_remove": {
"count": 8364
},
"cooking_recipe": {
"count": 10557
},
"email_querycontact": {
"count": 6477
},
"lists_createoradd": {
"count": 9027
},
"transport_taxi": {
"count": 5100
},
"qa_maths": {
"count": 3978
},
"social_post": {
"count": 14433
},
"qa_currency": {
"count": 7242
},
"email_addcontact": {
"count": 2754
}
}
}
}
This dataset card was automatically generated using MTEB